AI-Driven Hydrogen Catalyst Boosts Efficiency, Cutting Costs for Clean Energy Future
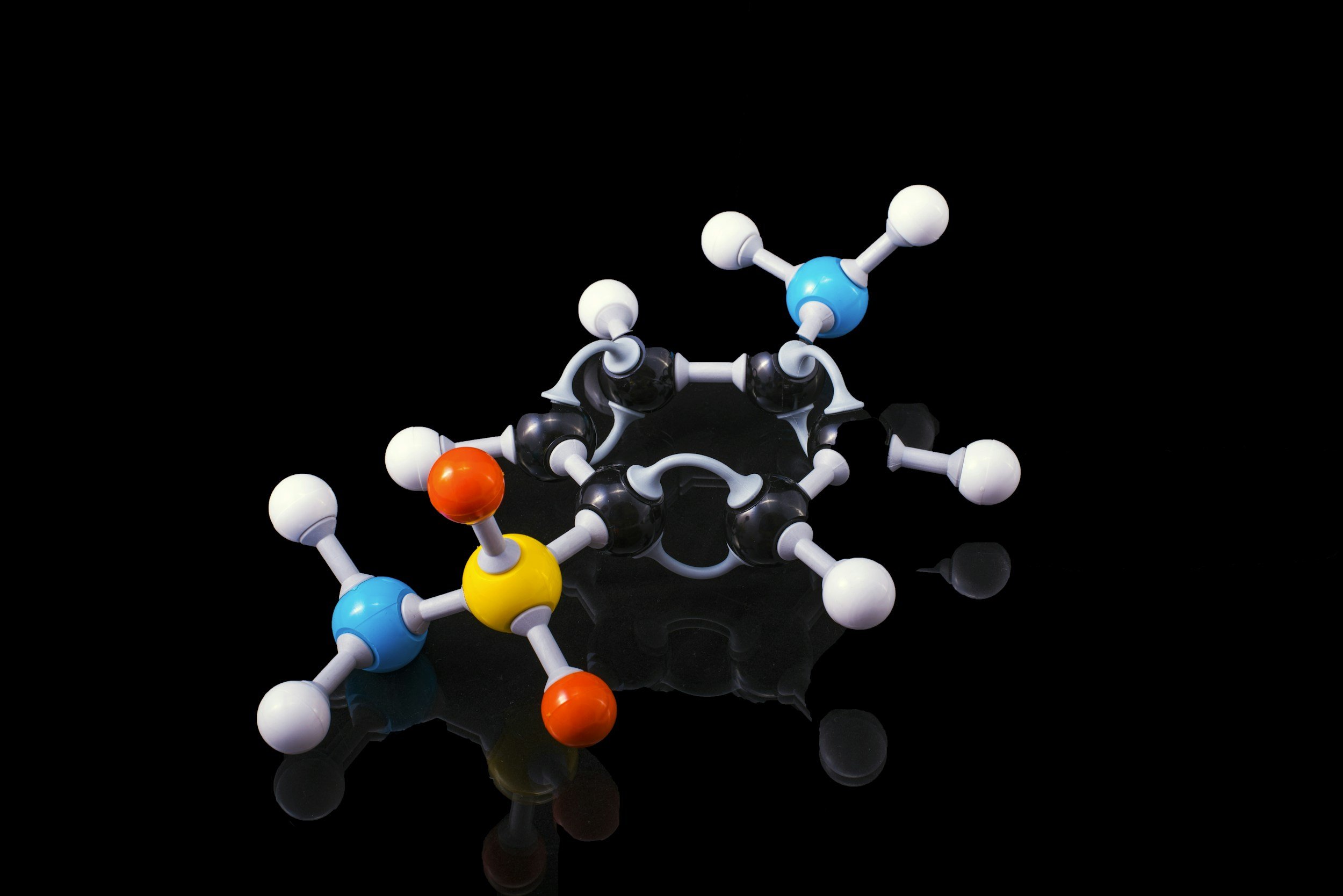
Image Credit: Terry Vlisidis | Splash
On April 25, 2025, ScienceDaily reported a groundbreaking advancement in hydrogen production, driven by an AI-guided “surface reconstruction” strategy. Researchers developed highly efficient catalysts that increase hydrogen yield while reducing energy use, a vital step toward affordable, carbon-neutral fuel. This AI-driven innovation could make hydrogen a practical clean energy solution.
AI’s Central Role in Catalyst Design
Artificial intelligence is the cornerstone of this breakthrough. The research team trained machine-learning models on high-throughput experimental data—large datasets generated quickly through automated experiments—to identify optimal catalyst materials. Unlike traditional methods, which rely on slow trial-and-error, AI analyzed patterns to predict compositions that enhance the hydrogen evolution reaction (HER), the process of splitting water to produce hydrogen gas. This approach accelerated the discovery of a new catalyst, cobalt phosphide modified with fluorine (CoP|F-20), which boosts efficiency and reduces reliance on costly materials.
The AI focused on “surface reconstruction”, a technique to modify a catalyst’s surface for better performance. By analyzing data, the AI determined that adding fluorine to cobalt phosphide creates phosphorus vacancy sites—specific areas on the catalyst’s surface that improve the HER. These sites allow the catalyst to produce more hydrogen with less energy.
[Read More: Extropic Develops Probabilistic Computing Chip for AI Efficiency]
Catalyst Performance and Durability
The AI-designed CoP|F-20 catalyst proved highly effective and durable. Laboratory tests in a three-electrode setup showed stable performance for over 300 hours in acidic conditions, critical for practical use. The catalyst was also tested in commercial-scale proton exchange membrane (PEM) electrolyzers, aligning with the U.S. Department of Energy’s 2026 goal of producing hydrogen at $2.00 per kilogram.
Traditional catalysts, like platinum and iridium, are effective but expensive and scarce. The AI-guided approach uses cobalt, an abundant and affordable material, modified to match or exceed the performance of noble metals. This shift reduces costs and enhances scalability, addressing key barriers to widespread hydrogen use.
[Read More: AI Kicks Away Bitcoin? The Power Struggle for Energy Resources]
Impact on Clean Energy
Hydrogen is a promising clean fuel, producing no carbon emissions when used. However, high production costs and energy demands have limited its adoption. The AI-driven catalyst lowers these barriers by improving efficiency and reducing reliance on costly materials. This could expand hydrogen’s use in transportation, manufacturing, and energy storage, supporting global climate goals.
Scaling this technology for commercial use requires further testing and investment. Electrolysis also relies on pure water, posing challenges in water-scarce regions. Addressing these issues will be critical to realizing hydrogen’s potential.
Research Credibility
Published in Advanced Energy Materials on April 3, 2025, the study was conducted at Tohoku University’s Advanced Institute for Materials Research. The findings were validated using advanced techniques like Raman measurements and operando X-ray absorption spectroscopy, ensuring robust data. Reports from Asia Research News and Open Access Government corroborate the study’s significance, reinforcing its credibility.
Source: Science Daily, Open Access Government, Mirage News
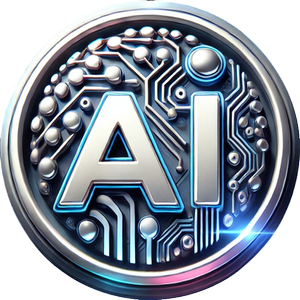
We are your source for AI news and insights. Join us as we explore the future of AI and its impact on humanity, offering thoughtful analysis and fostering community dialogue.